https://www.cohenveteransbioscience.org/wp-content/uploads/2024/01/cv.webp

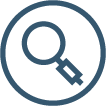
back to main menu
Brain Trauma
Brain trauma affects millions. Learn about traumatic brain injury (TBI) and post-traumatic stress disorder (PTSD) and how they impact civilians and Veterans.
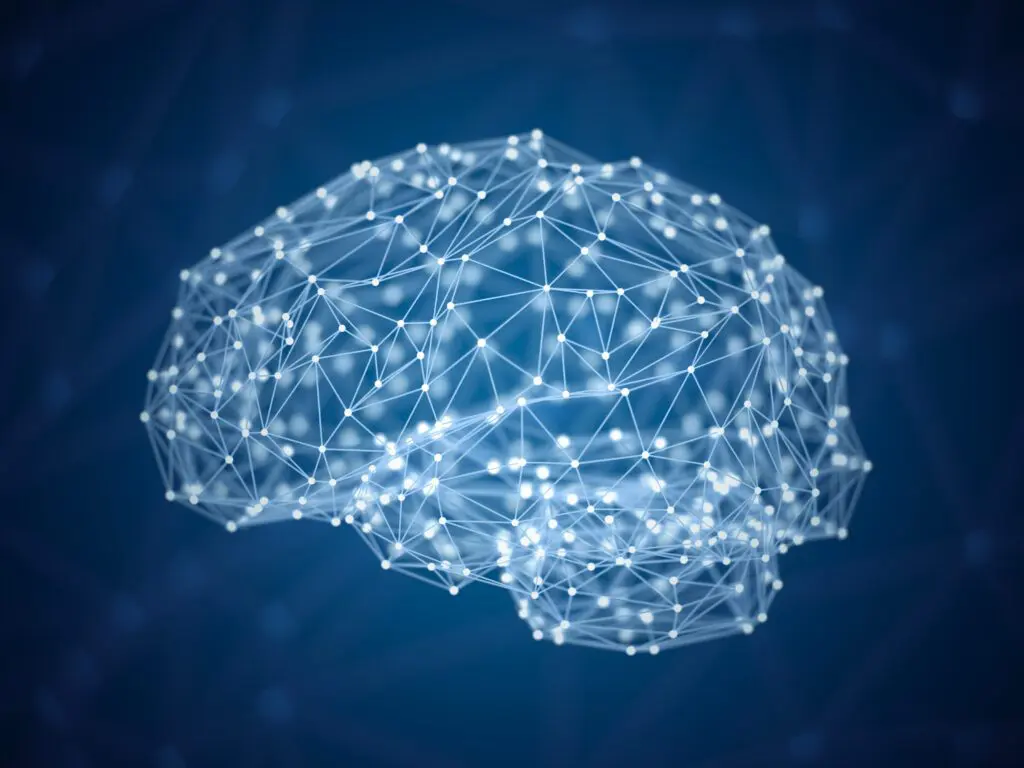
About Brain Trauma
Understand brain trauma (TBI/PTSD) and the current state of research.
Traumatic Brain Injury (TBI)
TBI symptoms, diagnosis, treatment, and the impact of our research.
Post-traumatic Stress Disorder (PTSD)
PTSD symptoms, diagnosis, treatment, and the impact of our research.
Brain Trauma & Veteran Suicide
Learn about suicide risk among military Veterans with brain trauma.
Join the TBI Action Alliance
A new Community Coordination model to accelerate a first generation of diagnostics and treatments for Traumatic Brain Injury.
back to main menu
Who We Are
Cohen Veterans Bioscience is a non-profit 501(c)(3) biomedical research and technology organization dedicated to advancing brain health by fast-tracking precision diagnostics and tailored therapeutics.
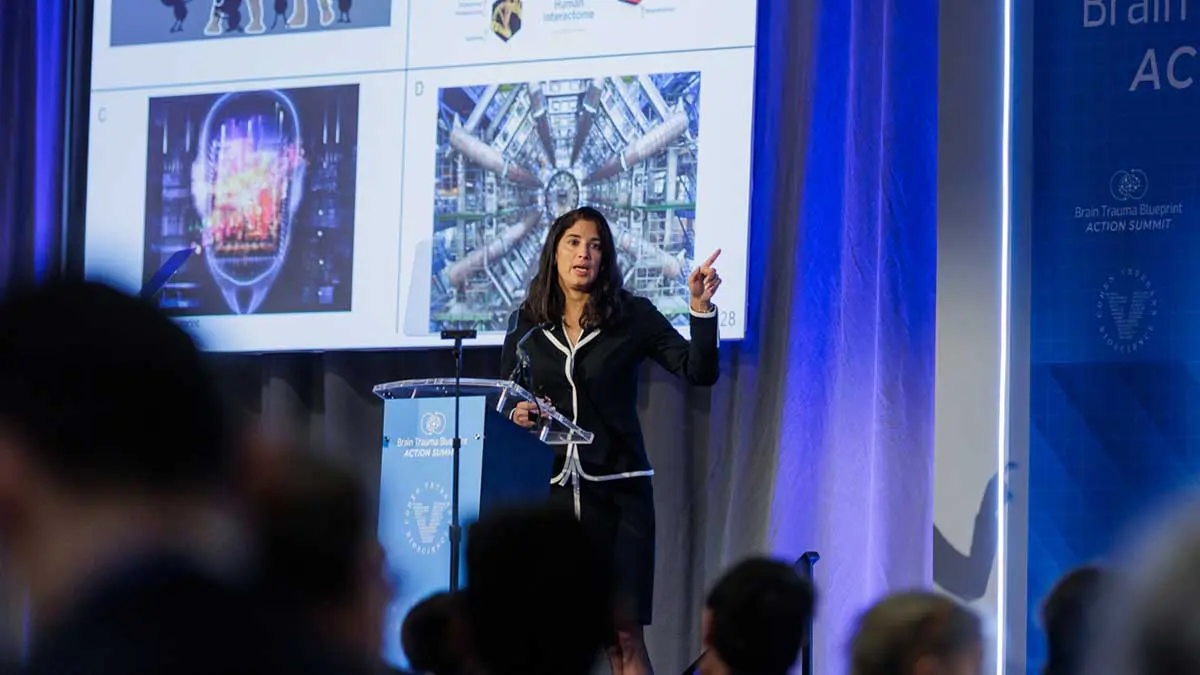
About Us
Explore our Mission & Vision to advance solutions for brain trauma.
Our Team
Meet the minds behind CVB.
Board of Directors
Meet our Board of Directors.
Veterans Advisory Council (VAC)
A voice for the Veterans community.
Patient & Veteran Perspectives
Read stories from people living with brain trauma.
Careers
Interested in joining the team? Explore careers at CVB.
Press Room
Connect with the experts at CVB.
back to main menu
What We Do
Our approach is to build enabling platforms with strategic partners and to adopt a team science approach to fast-track solutions in years, not decades.
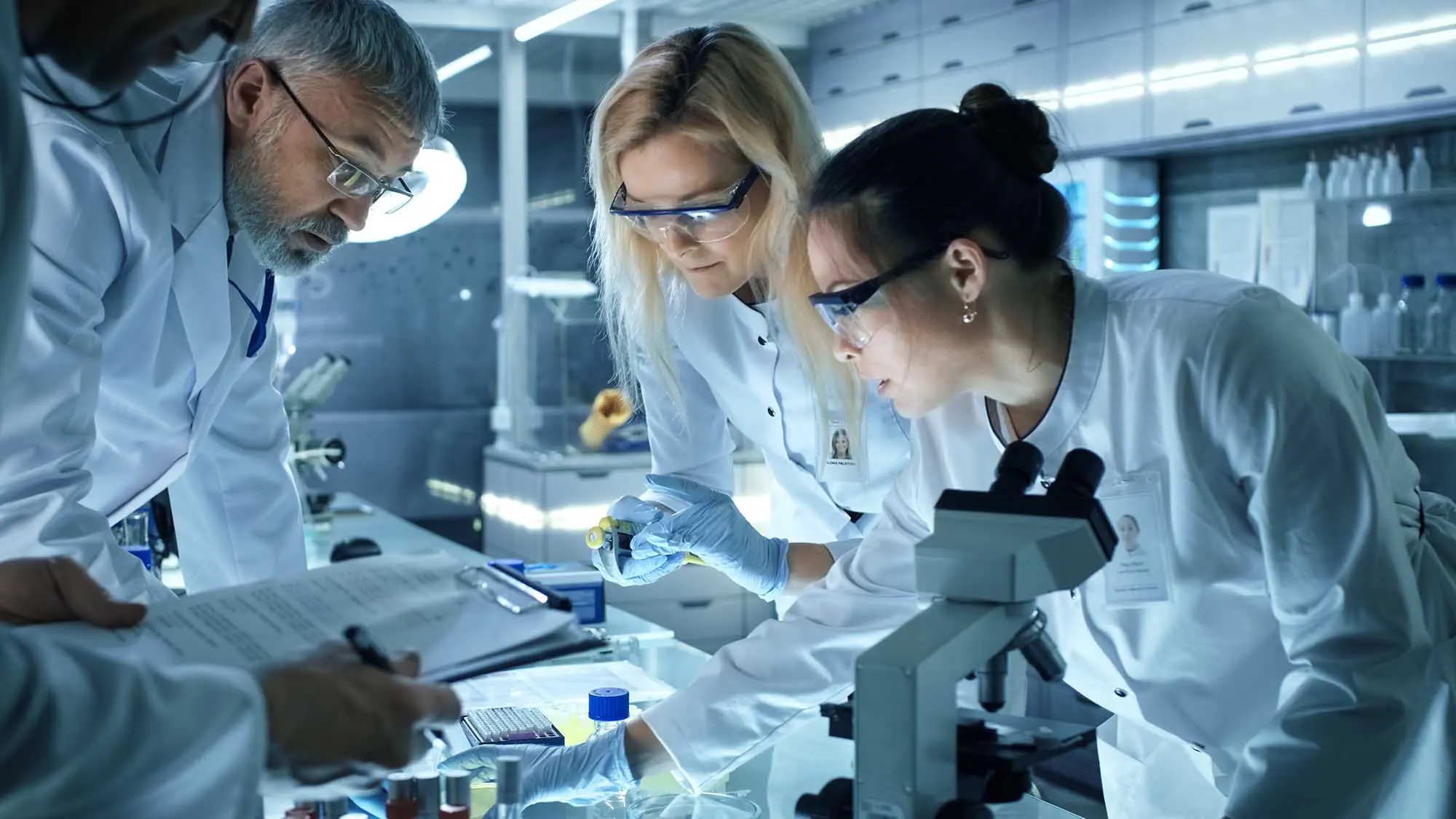
Research Programs
Fast-tracking diagnostics & therapeutics for brain trauma.
Advocacy Efforts
Advocating at the federal, state, and local levels.
Platforms
Helping high-impact research succeed.
Best Practices & Training
Driving quality & reproducibility in science.
Publications & Resources
Explore our research publications.
Veterans & the Military Community
Advancing our understanding of invisible wounds.
Clinical Trial Participation
Learn how to participate in clinical trials.
Leadership & Collaboration
Fostering a collaborative approach to research.
back to main menu
Join Us
Donate today to advance solutions for brain trauma. Together, we can advance research and improve lives.
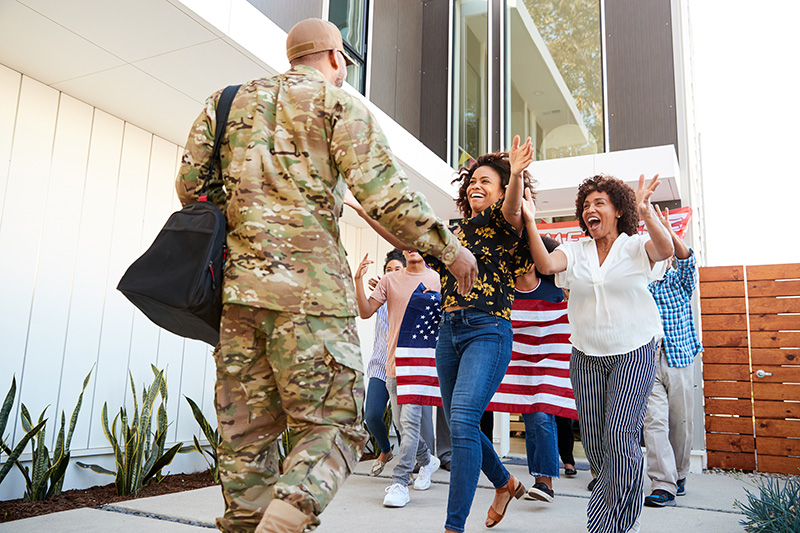
Sign Up for Our Newsletter
Get the latest updates in PTSD & TBI research.
TBI Trailblazers Volunteer Network
Share your story: how has TBI impacted you?
Contact Us
We look forward to hearing from you.
News & Events
Learn about the latest news & events from CVB.
In the News
View press releases and more.
Join the TBI Action Alliance
A new Community Coordination model to accelerate a first generation of diagnostics and treatments for Traumatic Brain Injury.